Loading Profile
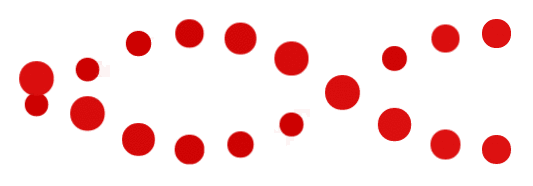
Orly Alter
Current Research
Orly Alter develops quantum mechanics-based multi-tensor AI/ML,1 which, as she experimentally validated, is uniquely able to discover accurate, precise, clinically actionable, and mechanistically interpretable predictors from small-cohort, noisy, and multi-dimensional, multi-omic data.2 Alter is a USTAR associate professor at the Scientific Computing and Imaging Institute3 and the Huntsman Cancer Institute at the University of Utah, a scientific advisory board member of the NCI-DOE Cancer Moonshot collaboration, and the CSO and a co-founder of Prism AI Therapeutics, Inc.4 As a genetics postdoctoral fellow at Stanford University, she invented the concept of the "eigengene" in a top 50 most cited PNAS paper of all time.5,6,7 Her Ph.D. thesis in applied physics, also at Stanford, was published by Wiley8,9,10 and is recognized as crucial to gravitational wave detection and quantum computing.11,12
Alter proved that her multi-tensor algorithms,13,14 known as comparative spectral decompositions,15,16,17 (i) always converge, and (ii) almost always into a mathematically unique model, from any data types, of any number and dimensions. She showed that her models (iii) comprehensively remove batch effects and capture known biology, and (iv) correctly predict previously unknown mechanisms.18,19 Her clinical trial validation of a pattern of DNA copy-number alterations in brain tumors solved the 70 year-old problem of correctly predicting a patient's response and survival from their genome,20,21,22 and demonstrated that her predictors are (v) the most accurate and precise, (vi) clinically actionable in the general population based upon as few as 1923 patients, and (vii) consistent across federated studies and over time. Recent experiments additionally demonstrated that the models (viii) correctly predict drug targets to sensitize a tumor to treatment.24 Alter discovered and validated multi-omic predictors, in cancer25 and other diseases, in public data, establishing that her AI/ML is uniquely suited to personalized medicine.